AI Future
Artificial Intelligence (AI) is set to shape the future with unprecedented advancements, revolutionizing industries through breakthroughs in deep learning, natural language processing, and autonomous systems. As AI continues to evolve, it promises to drive innovation, redefine human interaction with technology, and unlock new possibilities across every sector.
The Foundations of AI
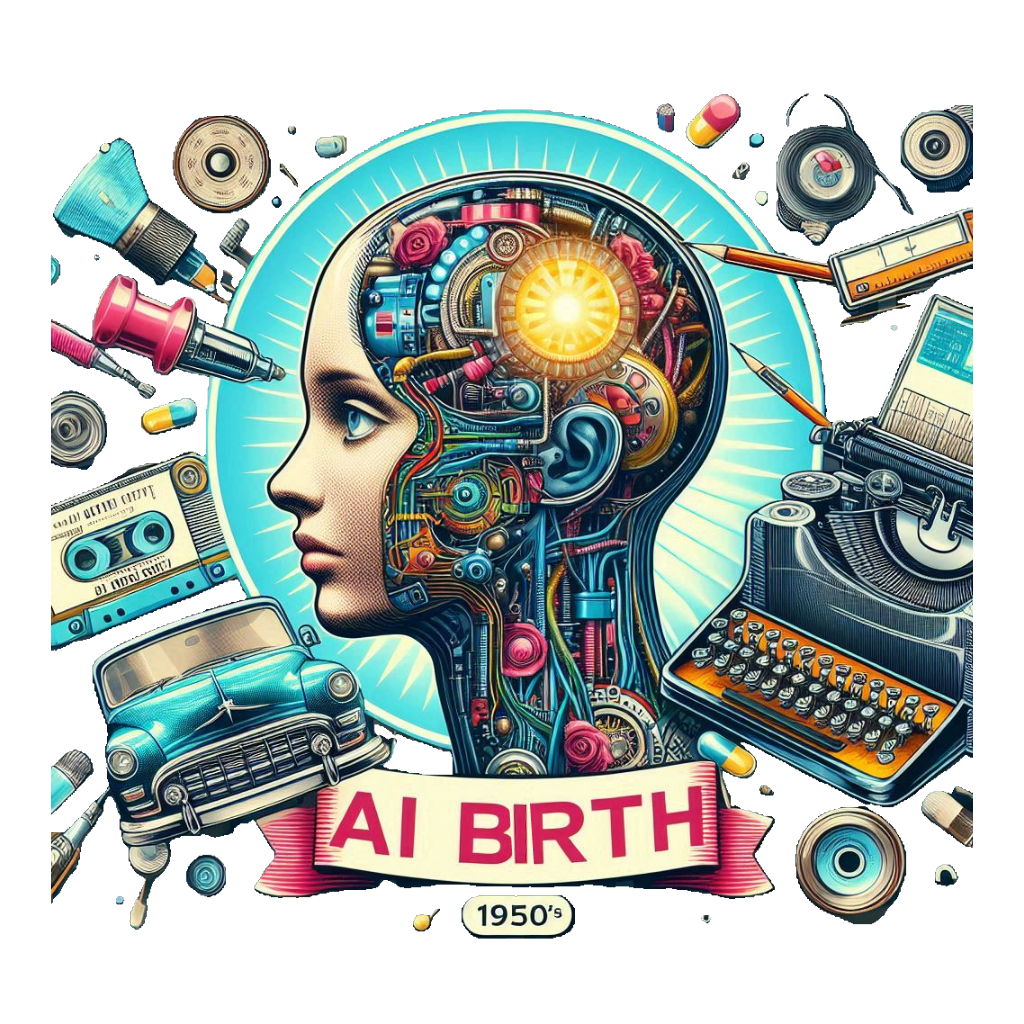
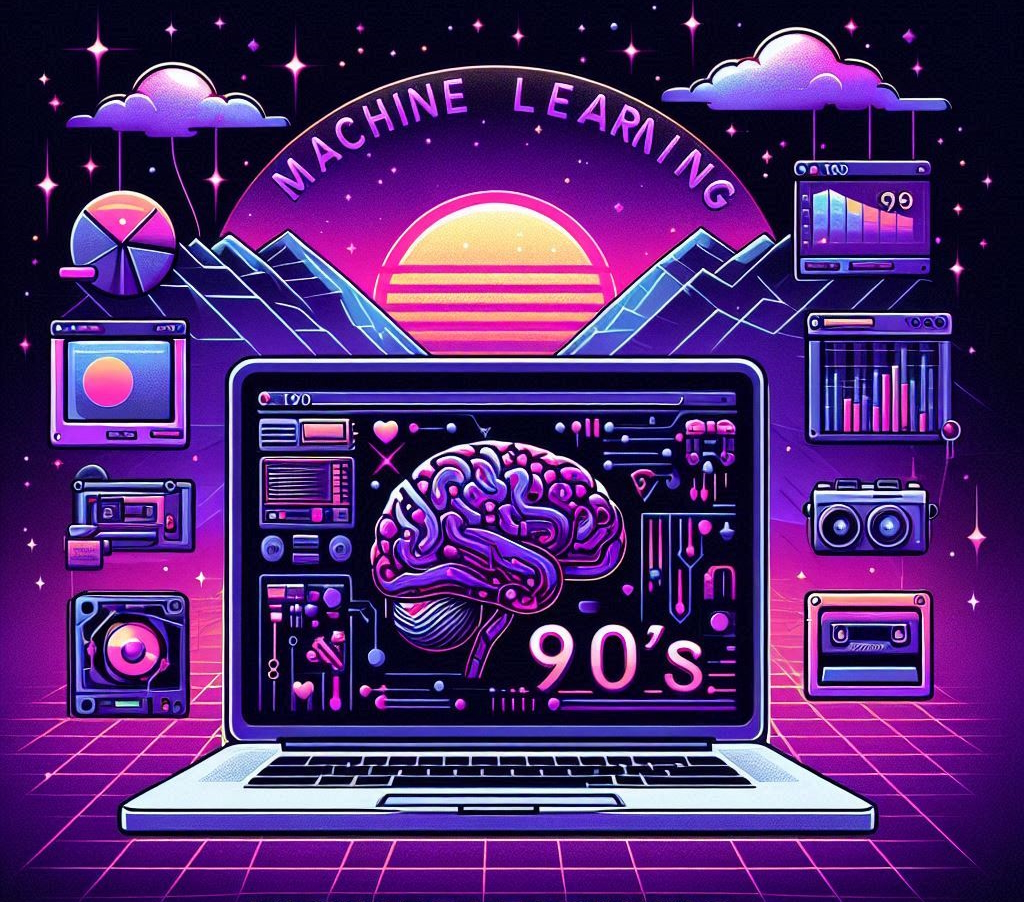
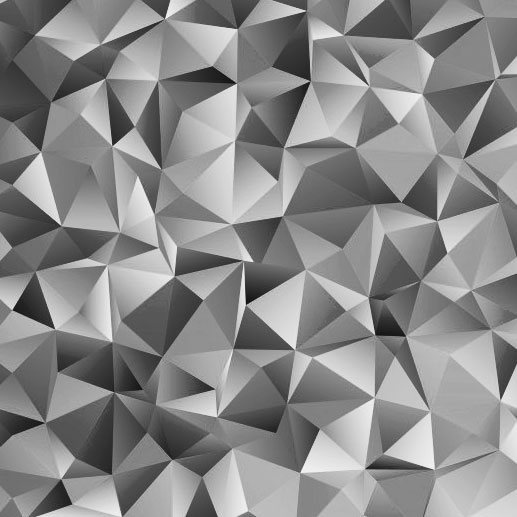
Conceptual Origins: The journey of artificial intelligence began with theoretical ideas about machines being capable of reasoning and making decisions. Alan Turing, a British mathematician, is often regarded as one of the key figures in this foundational phase. In his 1950 paper, “Computing Machinery and Intelligence,” Turing proposed what would later be known as the Turing Test. The test was designed to determine whether a machine could exhibit intelligent behavior indistinguishable from that of a human. This work set the stage for future discussions on the nature of machine intelligence and laid the groundwork for what would later become the field of AI.
Early Visionaries: During the 1950s, several other pioneering thinkers began to explore the possibilities of creating machines that could think. Norbert Wiener, known for his work in cybernetics, explored how feedback loops could allow machines to adapt and learn from their environment, while Claude Shannon, a mathematician and electrical engineer, investigated the potential of digital circuits to solve logical problems, setting the technical foundations for computer science.
- The Dartmouth Workshop (1956): The concept of AI was formalized during the Dartmouth Workshop in the summer of 1956, which is widely recognized as the official birth of artificial intelligence as a field of study. Organized by John McCarthy, Marvin Minsky, Nathaniel Rochester, and Claude Shannon, this workshop was where the term “artificial intelligence” was first coined. The participants were optimistic about the prospects of creating intelligent machines within a few decades. They proposed that “every aspect of learning or any other feature of intelligence can in principle be so precisely described that a machine can be made to simulate it.” This bold vision attracted researchers from various disciplines and set the agenda for AI research for years to come.
Early Enthusiasm and Setbacks
- Initial Progress: Early AI research produced pioneering systems like the Logic Theorist (1955) and the General Problem Solver (1957), fueling optimism about AI’s potential.
- The First AI Winter: Overestimations of AI capabilities led to unmet expectations, causing funding cuts and diminished interest in the 1970s, a period known as the first “AI Winter.”
Revival Through Expert Systems
- The Rise of Expert Systems: AI research regained momentum with the development of expert systems such as DENDRAL and MYCIN, which used rule-based reasoning to emulate the decision-making of human experts.
- Commercialization: These systems found practical applications in various industries, including healthcare and finance, leading to renewed interest and investment in AI.
The Machine Learning Era
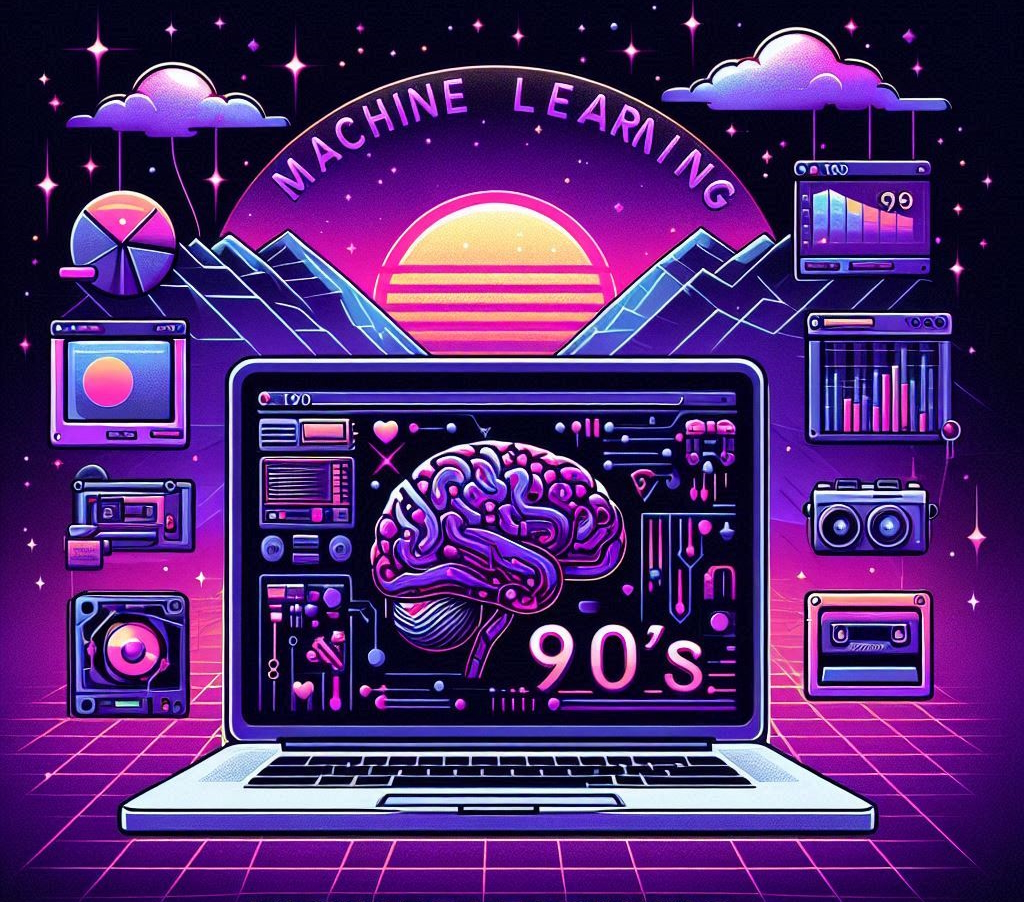
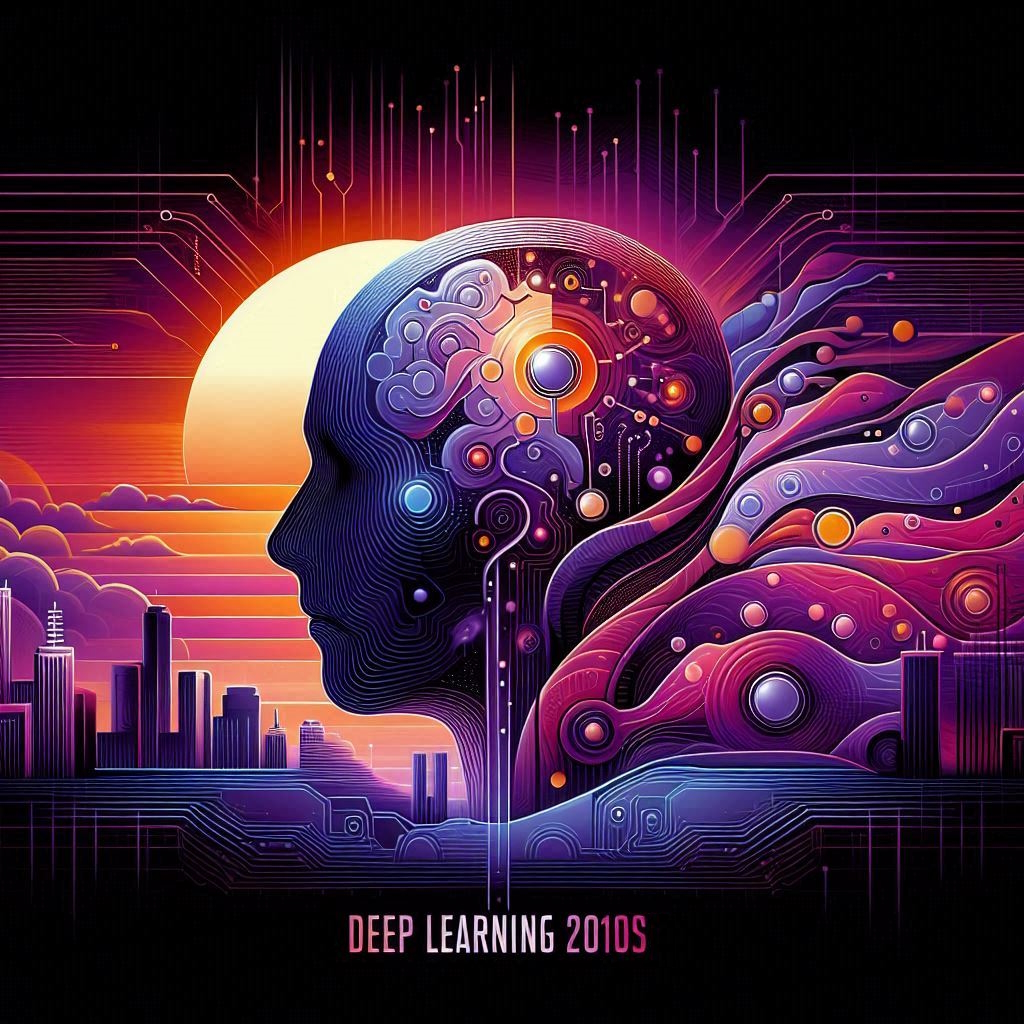
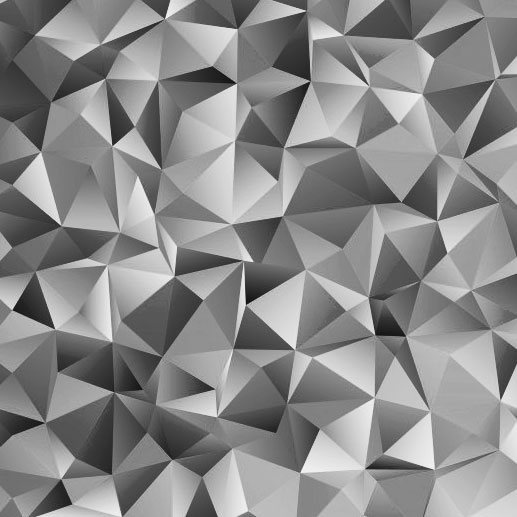
Shift to Machine Learning: In the 1990s, AI research began shifting from symbolic AI, which used rule-based systems, to machine learning. This transition was driven by the need for algorithms that could learn from data and adapt over time. The increased availability of large datasets and advances in computational power made it possible to develop more sophisticated learning algorithms.
Development of Key Algorithms: This era saw the rise of important machine learning techniques, including decision trees, support vector machines, and early neural networks. These methods improved the ability to make accurate predictions and classify data. Ensemble methods, which combine multiple algorithms to improve performance, also gained traction during this time.
Significant Milestones: A major milestone was IBM’s Deep Blue defeating chess champion Garry Kasparov in 1997, showcasing AI’s potential in complex tasks. Additionally, AI systems began to excel in specialized tasks such as image and speech recognition, demonstrating practical applications and increasing interest in machine learning technologies.
Commercial Impact and Growth: The 2000s saw the commercialization of machine learning, with companies integrating these technologies into products and services. Applications like recommendation systems for online shopping and predictive analytics in finance became commonplace. This commercial success spurred further research and investment, laying the groundwork for future AI advancements.
Advancements in Deep Learning
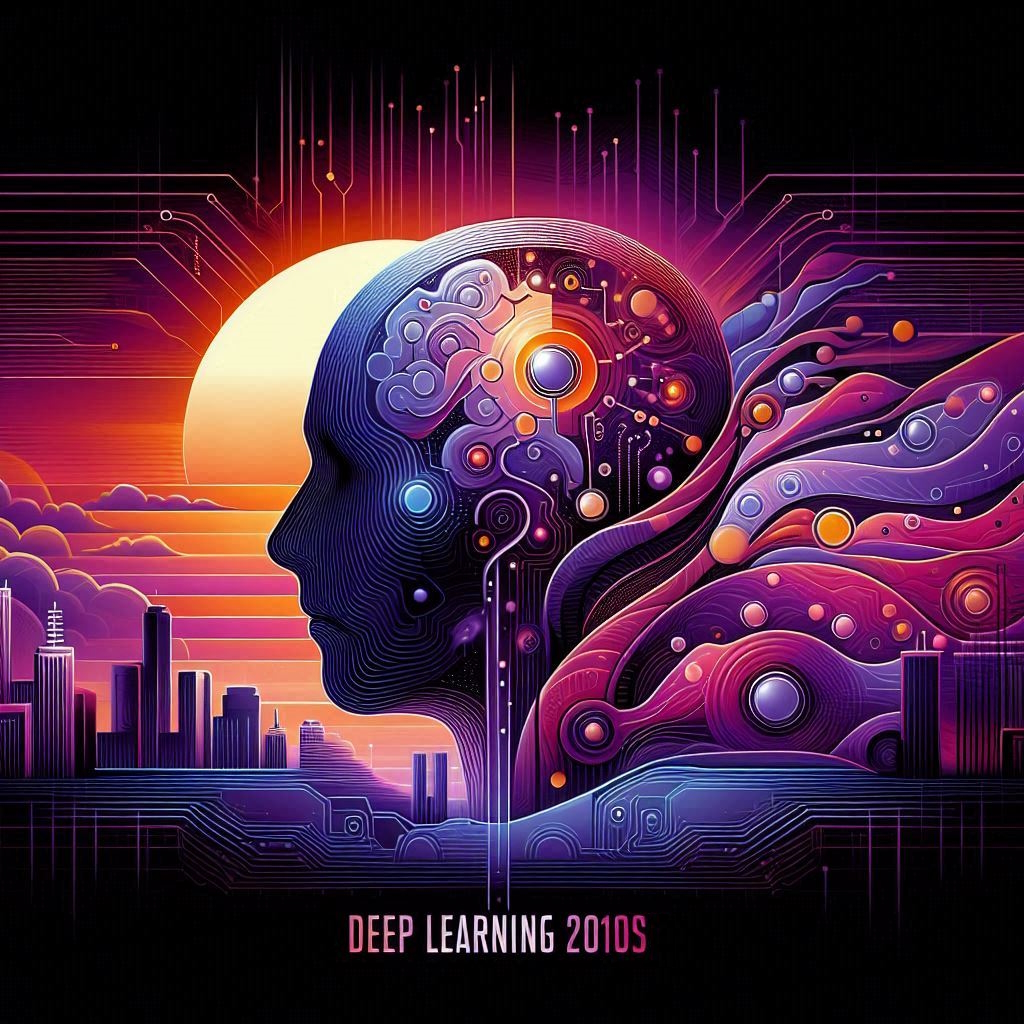
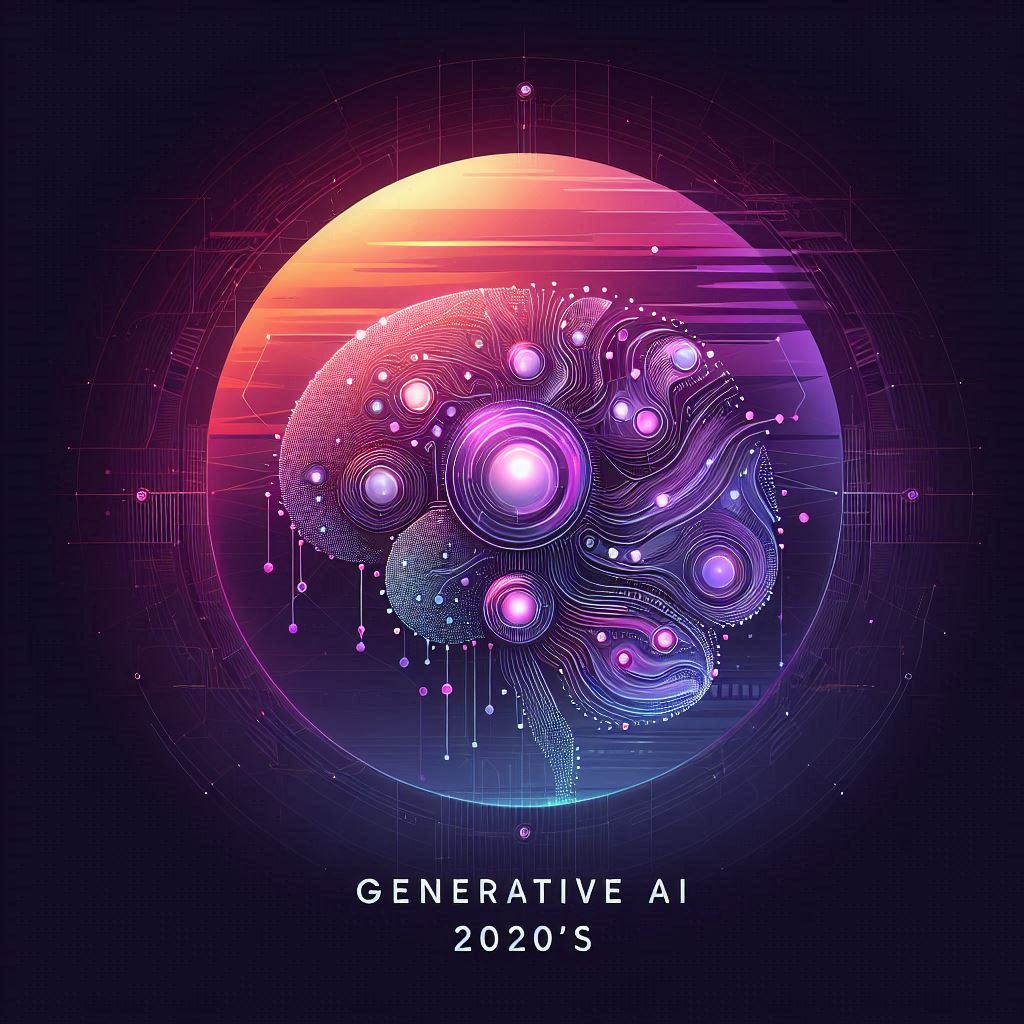
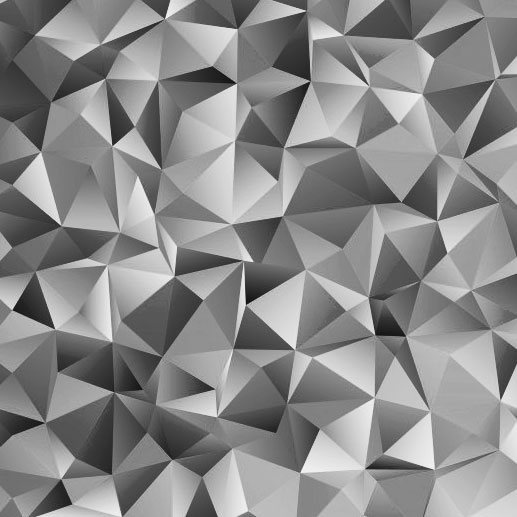
- Breakthroughs in Deep Learning: The development of deep learning, characterized by neural networks with multiple layers, revolutionized fields such as image and speech recognition.
Advancement of Neural Networks: The 2010s saw deep learning rise to prominence, with neural networks featuring multiple layers—known as deep neural networks—becoming central to AI advancements. These models excel in tasks such as image and speech recognition by learning complex features from large datasets, significantly improving performance and accuracy.
Impact and Applications: Deep learning has revolutionized various industries, leading to the widespread adoption of technologies like voice assistants, autonomous vehicles, and personalized content recommendations. These applications leverage deep learning to deliver more precise and efficient services, reshaping user experiences and business operations.
Future Directions: Research in deep learning is focused on enhancing model efficiency and interpretability. Innovations such as transfer learning and efforts to reduce computational costs are driving the field forward, promising new developments and applications that will further impact technology and society.
The Emergence of Generative AI
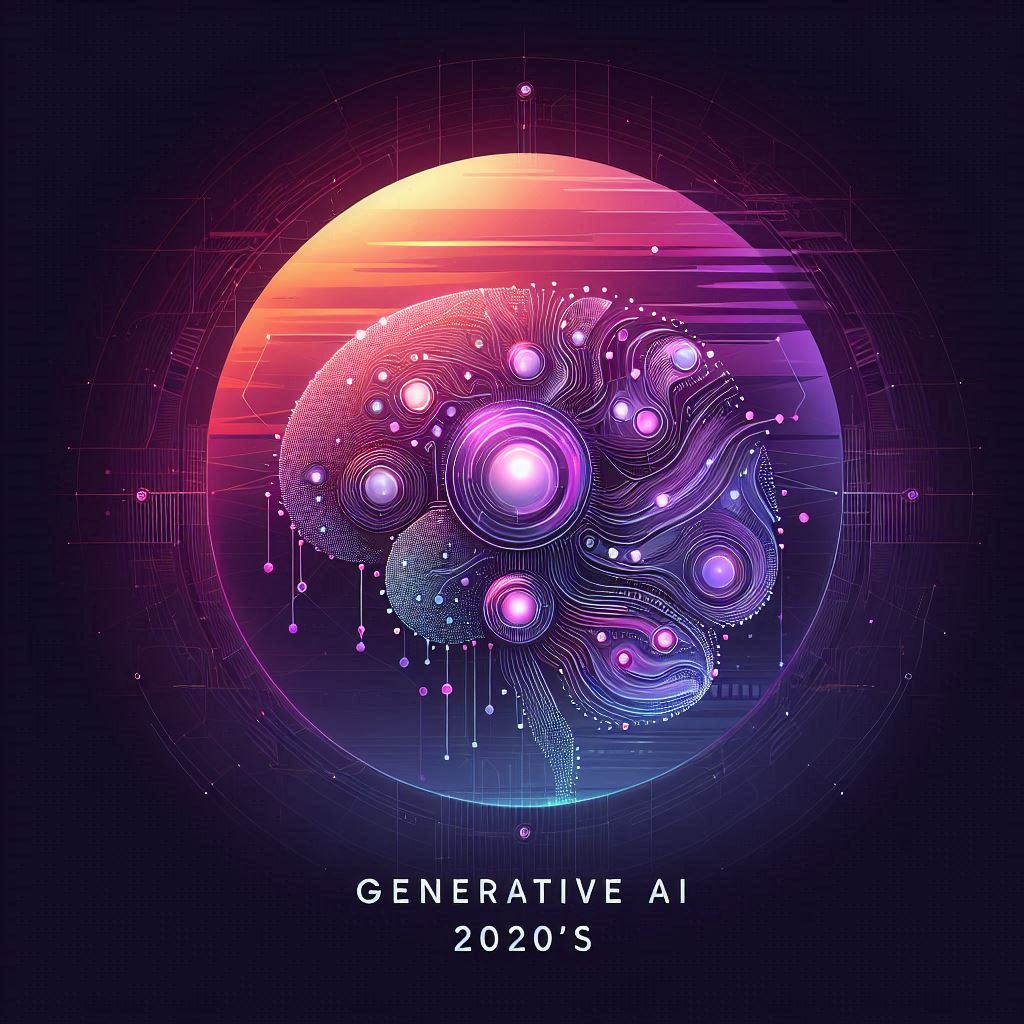
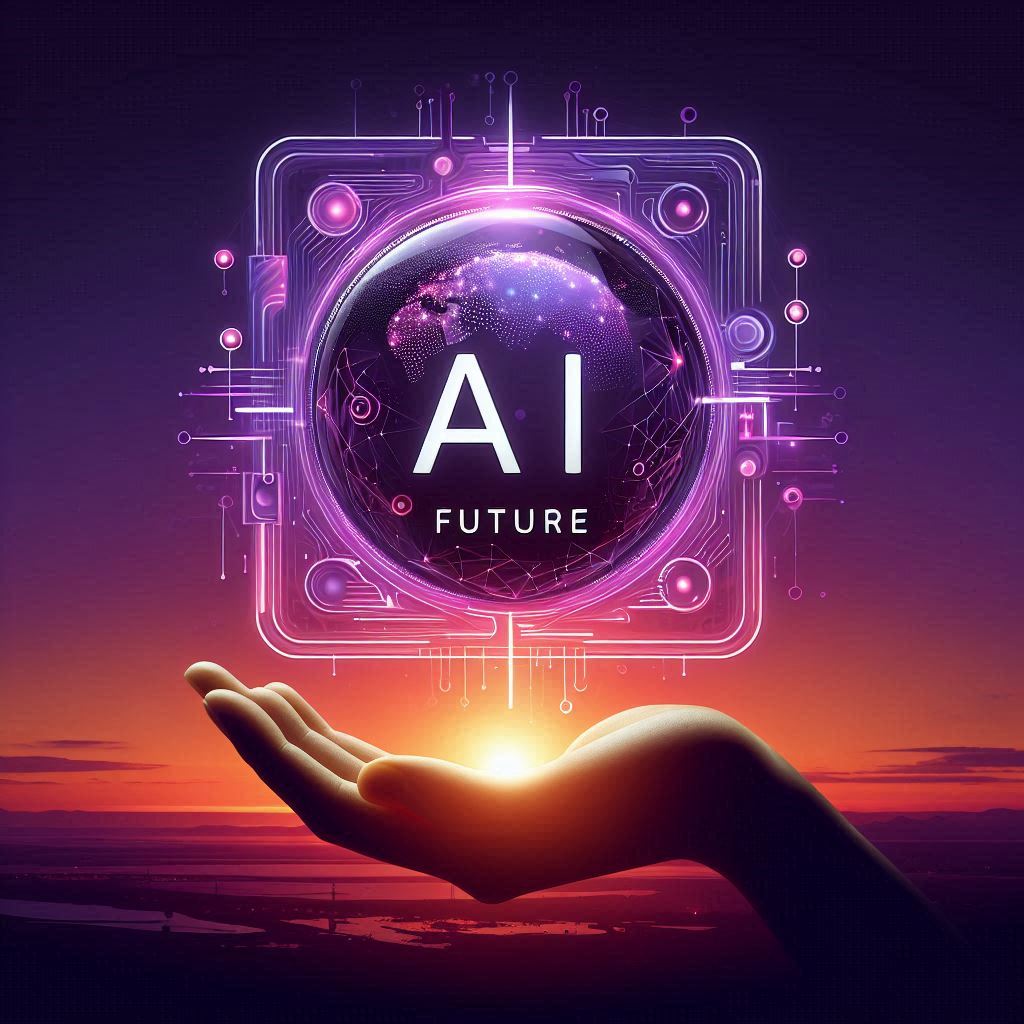
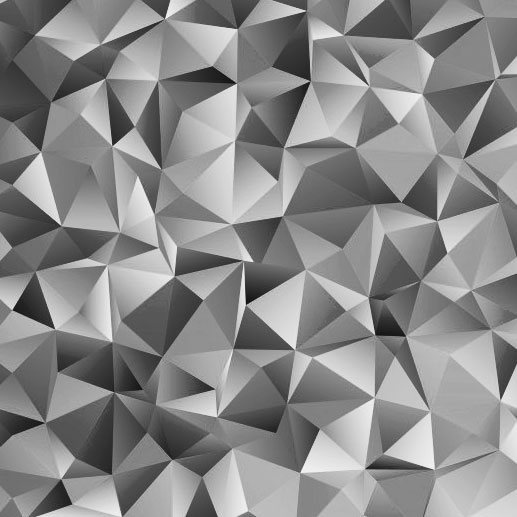
Generative AI Models: The 2020s have seen significant advancements with generative AI models like OpenAI’s GPT-3 and GPT-4. These models excel in producing coherent and contextually relevant text, making them highly effective for a range of applications including writing articles, creating content, and generating creative works. Their advanced language processing capabilities have set new benchmarks for AI in understanding and generating human-like text.
Broad Applications: Generative AI is increasingly influencing diverse fields. In content creation, it assists with drafting articles, developing marketing materials, and even writing stories. The technology is also used in design for generating graphics and artwork, and in entertainment for creating scripts and interactive experiences. Additionally, it powers conversational agents and virtual assistants, enhancing user interactions with more natural and responsive communication.
Ethical Considerations: The rise of generative AI has raised important ethical issues. Concerns include the potential misuse of deepfakes for misinformation, the implications for intellectual property in creative industries, and broader societal impacts such as job displacement and data privacy. These challenges underscore the need for responsible use and regulation of generative AI technologies.
Future Directions: Research in generative AI is focused on improving model performance and addressing ethical concerns. Innovations in multi-modal AI, which integrates text, image, and audio data, promise to expand the capabilities and applications of these technologies. Efforts are also underway to develop guidelines and safeguards to ensure that generative AI is used ethically and responsibly.
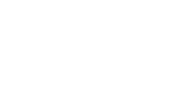
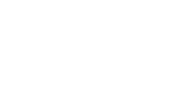
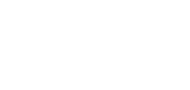
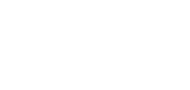
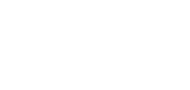
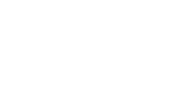